As technology proliferates it is clear the world of intelligence analysis is getting more democratized. But do we know how much? And are defense leaders reacting to it in terms of personal education and contracting reform? The Ukraine war features open source intelligence activities such as NASA satellites used to detect artillery fire and impacts and painstaking compilation of social media photos to track equipment losses. Such efforts are not entirely new; the French commercial SPOT IMAGE satellite was used to image troop movements in 1990 during the run-up to Operation Desert Storm. But there is a sense that, particularly in the processing and analysis of data, the democratisation trend is accelerating. Innovation now more often concerns software rather than hardware and is largely a private sector, or even private individual affair.
Falling Behind?
One anecdote demonstrating this shift is the Boeing corporation’s 60-year arc from creating the B-52; one of the 20th century’s most iconic and valuable weapon platforms, to presiding over the fiasco of Railhead. The latter was a post 9/11 software development effort to assist analysts in searching the American TIDE counter terrorism database. Railhead cost $500 million by 2008 and was bungled so badly it couldn’t even execute a Boolean search using terms like “and”, “or”, and “not”. Of course, in 2008, most of us were using Google which was powered by the PageRank algorithm, a Stanford graduate school project from the 1990s. Academic research, transferred to a startup, had lapped a well-funded government contractor with decades of experience working a critical target area.
Today many governments seem particularly behind the curve in deployment of Artificial Intelligence and Machine Learning (AI/ML) capabilities for national security tasks. Broadly speaking, AI/ML refers to a host of computer systems that can approximate human cognition and are useful for automating certain tasks like classification and prediction. Some news articles tracking developments in image processing give intriguing implications of AI/ML for intelligence fusion and insights into the raw power that open-source solutions can potentially provide for a military.
A South China Morning Post article attracted attention in the national security social media community because of the assertion that China had developed an AI/ML algorithm that successfully tracked the USS Harry S Truman, an aircraft carrier, via remote sensing data. The execution of an operational concept is notable, from a perspective of being news, but the amount of surprise and concern exhibited by some on social media, news, and online message forums seemed to indicate a lack of familiarity with the current state of either report sensing or AI/ML.
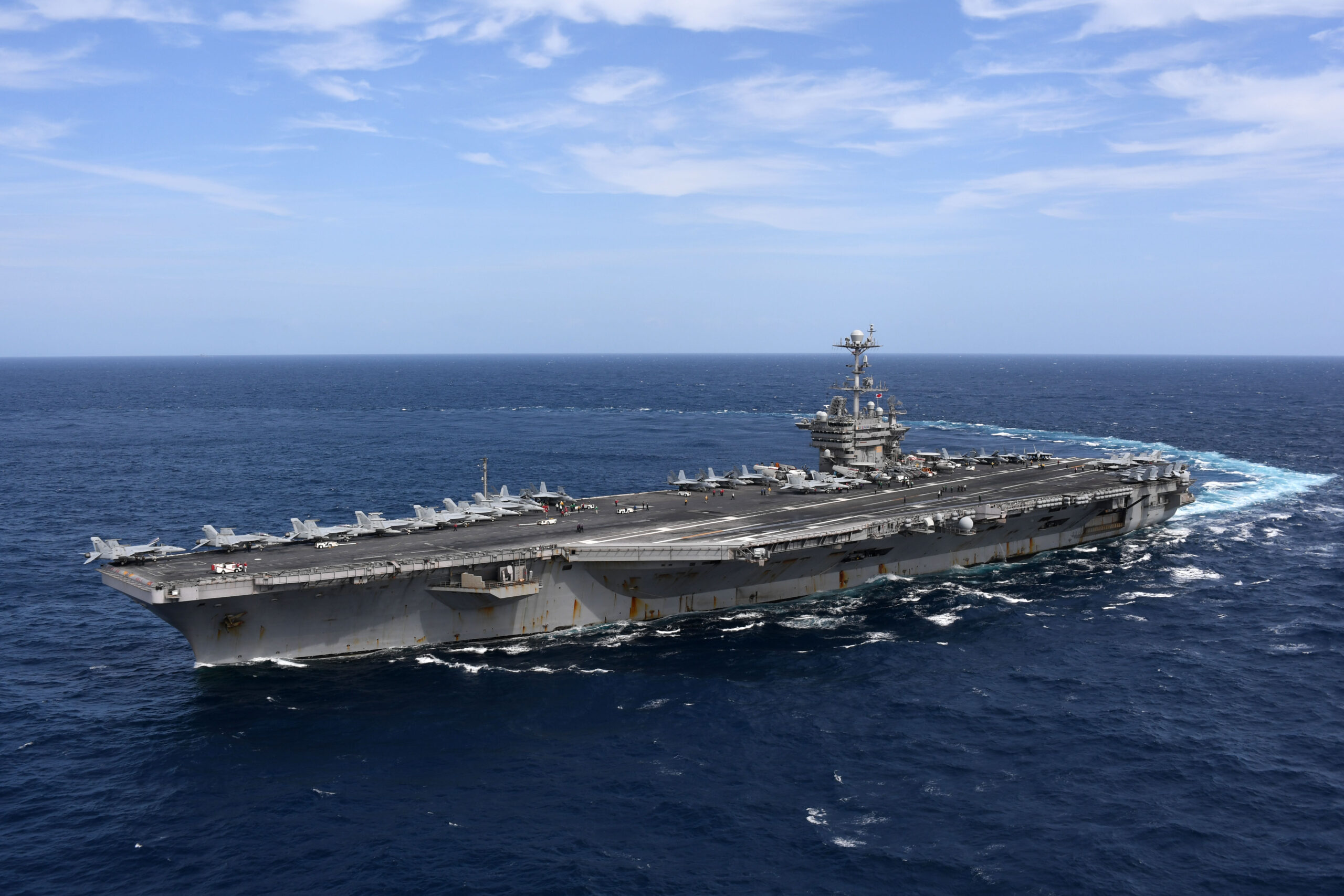
The development was not as dire as some portrayed it. First, open source reporting shows that China has had, since at least 2015, a wide aperture (400 by 400 km per frame) electro-optical overhead imaging platform with a spatial resolution of 50 meters, far less than the hull length of a Nimitz class carrier’s 331 meters. Perched in a geosynchronous orbit, the Gaofen-4 has a steady view of the ocean off China’s east coast. Creation of an AI/ML algorithm to do image recognition on an aircraft carrier afloat would have been a grad school level problem in 2015. Second, the idea of using convolutional neural networks (CNNs), which excel at image classification, to process satellite imagery and aid in tipping and cueing, was being discussed in open source literature at the US Air Force Institute of Technology as early as 2017. Today, an inspired high schooler could accomplish the task, perhaps using a CNN framework built into an open-source AI/ML software library like Tensorflow.
Fishing for Intelligence
Global Fishing Watch, a nonprofit organisation, presents another maritime use case for AI/ML to aid situational awareness. Last year on World Ocean Day the group unveiled a Global Fishing Watch Map to assist in tracking ‘dark vessels’, mainly fishing vessels, who turn off their Automated Identification Systems (AIS) to evade detection. While there are legitimate reasons for ships to switch off, such activity is often associated with illegal fishing or oil smuggling.
Global Fishing Watch wanted to correlate signals. AIS signals provide identity resolution on vessels such as name and registration while Synthetic Aperture Radar (SAR) returns can geolocate ships even when AIS transponders are off. The European Space Agency’s Two Sentinel-1 satellites provides the SAR data, collecting radar returns in strips 250 km wide as they orbit the Earth around 14 times a day.
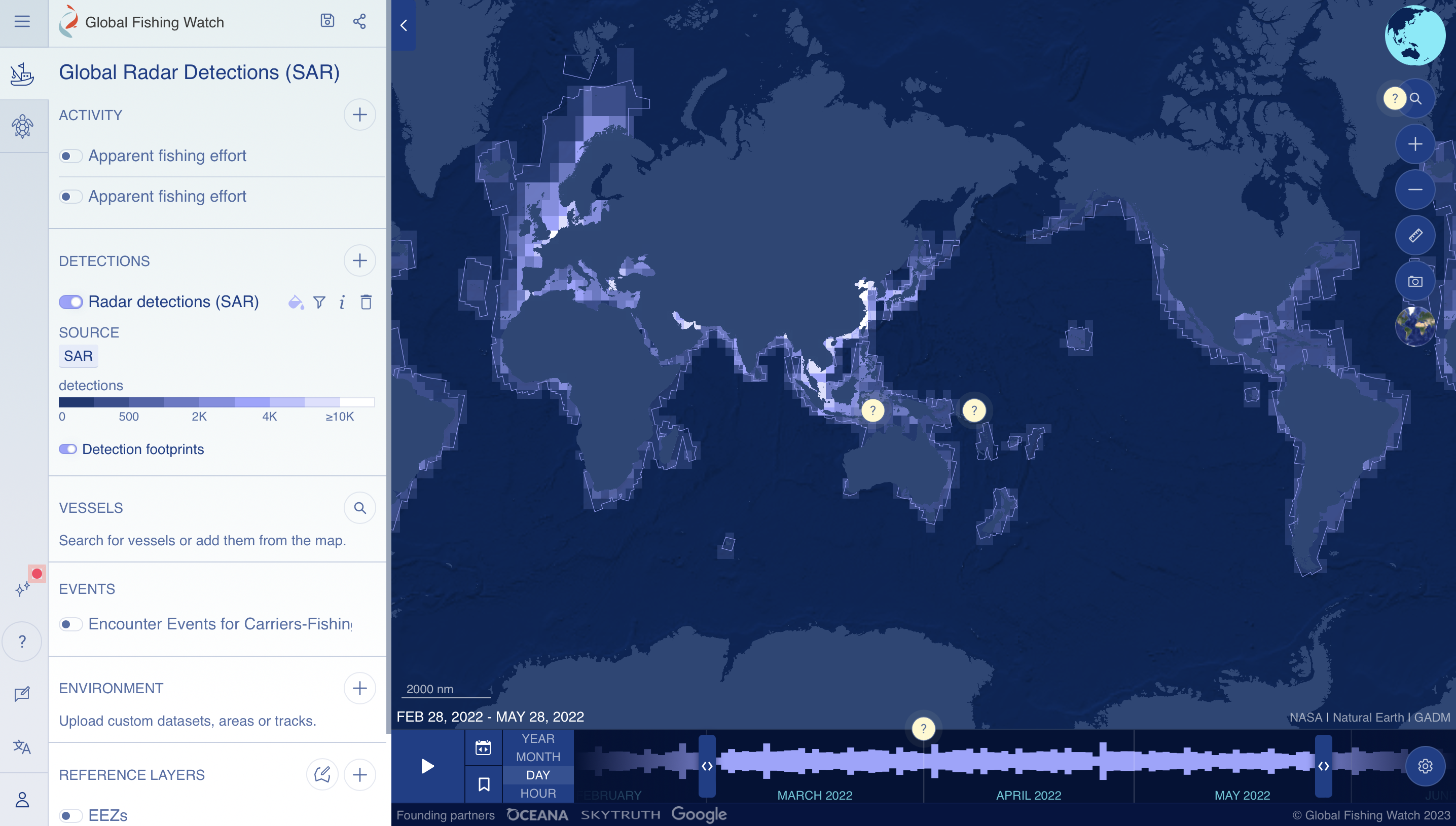
Correlating these AIS, and SAR datasets is not trivial. The nonprofit explained in a press release “by analysing the entire archive of Sentinel-1 radar imagery, Global Fishing Watch has made 20 million detections of sea-going vessels greater than approximately 10 meters in length—and matched these detections to 100 billion GPS points from vessels broadcasting their position on the automatic identification system.” This is an archetypal ‘big data’ problem, requiring specialised software, hardware, and refined imagination, judgment, quantitative reasoning, and technical (coding) skills on the part of the humans who wish to solve it.
Global Fishing Watch addressed this problem by crowd sourcing it. The non-profit teamed with The Defense Innovation Unit, a group affiliated with the US Department of Defense, to solicit solutions as part of the xView3 competition announced in 2021. 1,900 registrants from 67 countries offered solutions, vying for $150,000 in prize money. Five registrants won the cash, the biggest chunk going to Eugene Khvedchenia, a Ukrainian who goes by the moniker BloodAxe. XView3 was not Khvedchenia’s first competition. He is listed as a top 100 competitor on Kaggle, a website that hosts data science competitions, and as of 2022 held the number 1 ranking of 37,000 competing data scientists on the competition website signate.jp. He solved xView3 with an ensemble solution that combined methods, including a predictor that processes imagery two pixels at a time, allowing it to eke out every bit of sensor resolution from the Sentinel-1 radars, getting small ships that barely register with the sensor.
Many teams worked together to submit solutions to XView3 and were beat out by a singleton and graduate of the Odessa State Academy of Refrigeration. In a final twist to the story, BloodAxe’s ensemble AI/ML solution incorporated and modified a medical imaging processing system called CircleNet, which was designed at Vanderbilt University Medical Center to identify the ball-shaped glomerulus, a type of tissue in the kidney.
The Rise of the Citizen Hobbyist Expert
Khvedchenia/BloodAxe is still doing data science work; other code he has written is being used to crowd source battle damage assessments in Ukraine. He maintains a list of Russian domains he assesses as good candidates for crowd-sources Distributed Denial of Service (DDOS) attacks, and one feels he is probably up to other missions for his country as well. His mother, who was temporarily a refugee in Spain, runs an Etsy Shop stocked with wonderful Amigurumi dolls. One can see from whence he gets his attention to detail.
The XView3 vignette is powerful on its own and as a contrast to formal AI/ML investment approaches. Intelligence, Surveillance, and Reconnaissance (ISR) support to operational access requires sensors with the attributes of access, capacity, resolution, and persistence. Changing an ISR platform’s access (getting a sensor closer to a target), or its persistence- (its ability to ‘stare’ at an area of interest) almost always requires mechanical modifications, perhaps modifications that aren’t feasible. However, the Xview3 competition dramatically improved capacity and resolution of satellite sensors that are essentially impossible to physically upgrade. The cost was $150,000 plus program management expenses. In contrast, the U.S. Department of Defense spent approximately $50 million on initial rounds of Project Maven, a set of computer imaging contracts.
Many companies now offer AI/ML solutions to governments and most projects are much smaller than Maven. While there is BloodAxe-quality at firms small and large in this space, some of the offerings are essentially blog post tutorials and repackaged regression analysis masquerading as ‘advanced analytics’ and marketed by former insiders who understand the particulars of winning government contracts. The story of Railhead and Google PageRank remind us that governments can buy lemons, and expensive government projects can be lapped by private sector (or even private individual) developments. National security practitioners and thinkers should ask if governments are getting their money’s worth from AI/ML, and if they are developing the right leaders to acquire and manage these capabilities.
AI/ML investments can improve sensor detection rate, as with Global Fishing Watch’s project, computer imaging, as with Maven, signals intelligence, or the processing of unstructured text for analysts. Some business processes and logistics efforts are amenable to AI/ML. Flexport, a commercial logistics firm, uses AI to more efficiently handle customs paperwork. The project managers and acquisitions officers responsible for these efforts need not be boffins themselves. They should, however, be comfortable with maths and the concepts developers use to prepare, test, and evaluate the AI/ML models. They should be smart enough to be savvy consumers of the services, and confident enough to push back when a proffered solution is unacceptable. Such positions require a blend of technical and domain expertise, and solutions may differ from organisation to organisation.
So, what now?
Contracting reform, like intelligence reform, is a never-ending challenge for many governments including that of the United States. One part of the solution is to continue to grow leaders who are familiar with both the mission space and the technology, and who can differentiate fact from fiction and a revolutionary capability from an uninspired graduate school project. Today we are awash in data and companies willing to profit, justly or otherwise, from our desire to leverage it for business purposes. If defense leaders approach this situation shrewdly, they can have a huge impact, perhaps by contracting the next BloodAxe, or even recruiting him into government service. But if acquisition approaches are muddled, buyers run the risk of getting Machine Learning models that do not work, or are, in the phrasing of one tech consultant, and others little more than but “three linear regressions in a trench coat.”
Andrew Marvin
Andrew Marvin has served as a Field Artillery and Military Intelligence Officer in the U.S. Army and a consultant advising the government on national security issues. He currently serves as an Operations Research Analyst for the Department of Homeland Security. His views are his own.