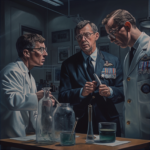
In this episode, Frosty and Peter Roberts talk to Dr Steven Meers from DSTL about Artificial Intelligence (AI).
Pete and Frosty discuss what AI research means in a military context. Steven talks through all the different ways AI can be used to benefit the military. He also dispels many of the myths around what AI is, what it can do and what its weaknesses are.
If you want to understand AI, this is a great place to get started.
Find the First episode on Human performance here
Transcript: (we use Descript to transcribe, apologies is there are a few errors).
So that’s the kind of a funny story, actually. So I was doing a PhD down at Southampton university. One day, I found a copy of the new. That had been left around in my, in the common room at the, at the, at the university. And on the back page, there was this advert for a job doing sonar analysis DG.
I thought, God, that looks amazing. I wanna, I’m gonna, I’m gonna chop my chop, my name in for that. Went through all the interview kind of first day in the office, really excited, walked into this like super secret secure office and found one of my PhD supervisors sat in there and it turned out the whole time I’ve been doing my PhD.
I could never find him on a Friday. And the reason was he was up at the S STL and taking some of like the exciting research that he was doing and applying it to real data sets and. I said to him, he didn’t leave a copy of the new scientist I get around in the common room. Did you case might have done?
Yeah. Yeah. So anyway, so yeah, that’s kind of where my career died was looking at sonar off submarines and over the years kind of data and. That’s data from submarines, data’s from satellites data from all kinds of sensors in the battlefield. That’s kind of been the, the sort of the golden thread in my career.
That’s led me now to work on AI, but
it’s something that’s evolved massively, right? I mean, you know, data sets, for example, people now talk about clean and dirty data sets. They talk about the number of data sets they can take in it. It feels almost like a, like a natural evolution. Isn’t it? It’s sort of moving from data science into.
Or is there a magic
leap? absolutely not. So I would say 80% of every AI project I’ve worked on has been about the data. You know, really digging into your data, understanding you know, what the data is actually telling you is really important. People get really excited about AI and it is, it’s a really exciting area.
I love the, the, the, the job. I do, but really AI is like the icing on top of the cake. The cake is made out of data
and that, and that’s really difficult, isn’t it? But I mean, I just remember, you know, from a nav career where there were, we should have had loads of databases to populate, you know, that would make systems work better, but we are not really very good at populating databases, but they are, as you say, absolutely crucial, aren’t they?
But when you move on, there’s sort of move from databases has then sort of gone through AI and will come to what we mean by AI, maybe in. But it sort of went through ML first, this machine learning thing first didn’t it does that has the same relationship with, is that just again different icing on the
top?
so I think this is one of the, the, the, the challenges around AI is it means different things to different people. I actually think it’s really simple in that for me, AI is about the ability of a system to learn, to complete a task. And if it doesn’t learn, it’s not intelligent. So often people kind of mix up all sorts of different things and say, oh, there, that that’s, that that’s AI.
Sometimes it might just be like a really simple kind of rules based automated kind of thing. Like a, I don’t know, like a robot in a, in a factory that’s making cars or something for me, that’s not intelligent. That’s just an automated system for me. The key thing that we are trying to build into our systems is the ability to learn.
So machine learning is a really. Strong example, artificial intelligence. That’s when most people say AI, I think they actually mean machine learning. And that’s where we’ve just seen incredible progress over the last 10 or 15 years. You know, you’ve probably seen it as much as, as, as I, but, you know Image classifies that can be better than the human eye at spotting things.
All kinds of games that people thought would never be won by a computer have now been you know, routinely, routinely beaten. So it’s just such a exciting era, but I think it is one where there’s an awful lot of excitement and a lot of misunderstandings about, about, about what it is and what it isn’t.
It is, cuz there’s that there’s an amazing definition around AI. Isn’t it? That, you know, some people say it has to be. And at that point, you start thinking, oh my God, what is being developed here? This is quite crazy. I mean, and, and therefore, you know, when speak to like Nina Collins in, in America, you know, professor of AI at the no war college, you know, and she will talk about, you know, this idea that we are decades away from that sentient moment.
But, but it, we’re not decades away from AI depending on how you define it. Right.
So AI. Like here today, we are all interacting with it, you know, dozens and dozens of times a day for me, you know, when I get up in the morning, I’m checking my news feed on my phone. There’s AI all over that. There’s AI in terms of how I interact with all kinds of of different systems.
So for me, yeah, artificial general. Is is really exciting. And when that kind of moment happens where we do get some kind of sentient machine that is gonna be a genuine, like existential moment for, for humanity. But what I don’t want it to do is to distract us from all of the really important applications of, of, of, of AI that we’re having to deal with here today.
And for me, particularly in the military domain, there are just so many really impactful areas where we. Apply current day AI techniques in a way that would really help war fighters. So,
so we’ll get this straight that we’re not gonna see, you know, the singularity, this is not what we’re working on. Right.
Is gray. Goo is the world being reduced to, you know, a sort of a matrix type thing that, I mean, that’s not what we’re talking about. We’re talking about something that has that, that we have around us every day and, and we’re talking. Militarizing those applications to, to produce better, stronger
signatures.
So when I talk about AI, I try and categorize it into three buckets. The the first bucket I use is what’s called narrow AI and almost all of the different applications of AI you will see in use today are what’s called narrow AI. That doesn’t mean they’re not incredibly powerful, but what it does mean is that they’re very highly specialized one trick ponies.
That can be fantastic at the task that they’re trained to do, but they can’t generalize. At the other end of the spectrum, you’ve got this kind of artificial general intelligence, which people typically define as being an algorithm that can do anything that a human can, any intellectual task that a human can.
And that’s that kind of moment, moment of Senti that you are, you are talking about. And as I said, that’s really important. We need to keep a really close eye on it. If we get that wrong, if we can’t control that system, we’ve got a, a huge, huge existential problem on our hands. But for me, the interesting bit is in the middle, I call that broad AI.
So it’s not a general intelligence that can do anything that a human can, but it’s not a one trick pony either. We’re beginning to see some examples of algorithms that are coming out of the universities out of some of the industry research labs now hit and here at the SDL. That I would call broad AI, which are AI that can begin to generalize, can begin to sort of take what it’s learned in one area and apply it to another area that begin to have some sort of common sense.
And we’re a long way off on that. And that will be a massive, massive leap when we, when we get there. But for me, I’m much more excited about broad AI than I am about artificial general intelligence, cuz that is a long way away as you say. So
in that, I’m just trying to picture it in my mind just to get it clear.
So broad AI. It’s not like a turbo charger. Right. Which you can stick in any car because it’s gotta learn something. Right. That’s that’s the, so it’s gotta learn something. So is it like a new engine management chip that you could throw in any car that would improve its performance or, or whatever, and actually you could take from a car and put into something else.
Is, is that what we’re talking about? So I would
say we’re still figuring it out. Yeah, it’s still it’s still research, but if you, if you think about how a baby learns, so. You know, children are incredible learners. If you’ve got young kids, you will have, you will have seen, I don’t know, they might learn that if they push a toy off their high chair, it makes a funny clashing noise.
And, you know, maybe they learn quite quickly that it breaks and to, to stop doing that, they would’ve learned that off, you know, maybe five or six times. And, and then they know, and then they can, then they can apply that if we had to try and teach a machine using. Narrow AI techniques to do that. We need to give it thousands of thousands of examples.
So I guess another example might be like a cat. You know, any baby can learn to identify a cat really quickly, and then you’ve shown a, a great cat rather than a black cat. They still know it’s a cat . If we have to train an AI to do that, it’s actually really hard. If you think about how many different rules you might come up with for what makes a cat, a cat, it’s got point of ears, it’s got whiskers, it’s got a furry furry tale.
There will always be some kind of variation you’ve not thought of. So for me, what I’m, what I think broad AI is about is trying to teach machines to learn a little bit more like humanly, trying to teach them.
Essentially yeah. A generalization
that you can take. Yeah, that’s right. Yeah. So there’s an amazing book called thinking fast and thinking slow by Danny Canman, who was I think he was a Nobel prize winning kind of scientist.
And he talked about in all of our brains, we have what he called system one. And what he called system two. Basically, we are really lazy and system one is like those heuristic that you mentioned how like real, like snap judgments, where we will see something and we will instantly might have to think about it.
We just, we just know it where system two is where we’ve really gotta think and that’s hard work. And so our brains like to be in, in, in, in insist of one. So you know, I. Where you might get to with all of this is that AI can do some more of that kind of sister, two thinking really kind of hard cognitive stuff, putting what it’s learned into context.
So, yeah, as I said, AI is a really important field, but it’s still, we’re still learning an awful lot. There’s an awful lot of research to do, but there’s also an awful lot of really mature technology that we can just apply to defenses problems. And so in DST, We’re in that really exciting space of trying to take the technology.
That’s been proven to work in other areas and really think how could that help the war fighter? How could that help save lives? How could that give more operational advantage to, to the UK? And I find that really exciting.
So, and, and it’s interesting, cuz I guess for the last five years, right? Every senior officer sprinkles, AI into every speech that they’ve talked.
And and saying, you know, this is the pixie dust. That’s gonna solve all our problems for the future. So what’s, DSTL what, what are you working on in AI terms? You know, give us a feel for what that, what that, what that’s like for
you?
So I think AI is one of the most ubiquitous technologies anywhere in defense, it’s not an end in itself, but it is a technology that can really transform lots and lots of different parts of defense.
So within. D SDL. We’ve got scientists and engineers that are conducting all kinds of different research activities from looking at AI for commander control applications and how we might be able to increase the operational tempo in a, in a military headquarters by injecting, you know, AI into small amounts of what headquarters does.
We are not talking about replacing humans. We’re talking about Augmenting some of the kind of manual repetitive tasks that a lot of humans undertake. Is that the sort
of thing that so narrow AI would probably be very useful for now? Yeah. So I think like Google maps that gives me, gave me three different ways I could come here and I made a decision from the airport to hear yep.
Based on, you know, my assessment of the threes gate, but it would’ve taken me. Get the map out and do all
that sort of stuff. So another example is natural language processing. So how you deal with, with large amounts of text you know, trying to quickly assimilate, you know, really long document or even worse, you know, a pile of really long documents.
How could we use AI to help the human commander more quickly kind of understand what’s going on? Around them? How can we help? Spend more of their time doing the thing that they’re really good at, which is understanding the operational context analyzing different courses of action, thinking through the operational risks and how can we use the machines to take away some of the like really.
Sort of du discovery work that, that, that, that can help them. So yeah, commander control, we think is a really exciting area. I mean, just the same, actually in the, the whole kind of intelligence surveillance and reconnaissance, the kind of the, the RSR space logistics cyber countering fake news.
That’s a really, really interesting one. So it is one of those technologies that is, is absolutely. Everywhere in terms of the, the research that D SCLs doing. So I’ll give you a few specific projects if you, if you’re interested in. So one team that D SCL runs is up at the center for intelligence innovation R whi and that’s where You know, there’s a lot of military analysts that are analyzing all kinds of intelligence feeds.
From around the world. They’ve been incredibly busy with with, with Ukraine recently, and in particular, they get an awful lot of satellite imagery that comes into Wied far more than they’ve got enough imagery analysts to, to train, to look at. So the D SGL team up at RF Whitten have developed a system called.
Spotter uses a narrow AI technique called convolution neural networks to analyze satellite imagery and to extract objects from that imagery so that the the analysts can quickly get to the imagery that they need to look at. So if we can triage all of the satellite imagery, that’s coming into RF, Whitten.
Prioritize them and say, these are the ones that need a human analyst to go away and look at. That’s the problem that, that, that spot is trying to solve.
Is that a bit similar to, I mean, there’s, the Ukraine has just seen open source intelligence, you know, on a massive scale in particularly in identifying, you know, fighting vehicles, movements, you know, large movements of troops, convoys, ammunition, dump.
And actually it’s open source that, that feels like it’s between. Is that similar to that? And is there then a difference in what the guys at Whitner doing and, and what’s been done, you know, in actually quite a small number of open source in those companies.
So we think the, the kind of traditional paradigm of intelligence analysis is flipping on its head.
For years it’s. A large number of classified sources of, of, of information that have been accessorized by some open sources. And as you rightly say, I think that paradigm has been, has been flipped. So, you know, using all of the different open source tools that are available to us, but then using our kind of incredibly capable kind of classified sources to corroborate, to get independent verification of what we are seeing in the, in the open source community.
And. You know, AI and, you know, the data science and the data engineering that sits underneath that is absolutely critical. If you’re gonna exploit that sort of fire hose of open source intelligence, you’re going to need some kind of AI or data science solution to do it.
You said you were gonna give us two advances.
So that was one. Yeah. Whi
so let me give you another one. So In November last year the SDL had a critical role in delivering the contested urban environment experiment in Portsmouth. This was a really large experiment with lots of nations all coming to the UK to explore how we could improve urban operations and particularly again, the, the, the, the ISR enterprise.
So at. The Q experiment tested over environment. Q we demonstrated a system called sapien. Sapien is a, an AI enabled intelligence surveillance and reconnaissance architecture. It allows us to bolt together, lots of different AI enabled sensors and then fuse all of those different sensors into like an autonomous sensor network.
And then again, using AI, choose what to expose to a human operator. So I want you to put yourself in the shoes of a security guard. That’s been sat in a hot dusty smelly port cabin, somewhere with a bank of CCTV monitors in front of them, maybe 20 or 30 CCTV monitors. How well do you think you would do in an eight hour shift trying to find the 30 seconds.
Really important information in that, in that, in that, in that, in that, in that, all that information, if you’re anything like me, you’d be awful at it. So that’s the kind of the problem that sapiens trying to solve. So at Q we used AI in the, in the sapien architecture, so that different sensors, whether it was a electro optic, like a camera or a thermal imager, An overhead UAV, or even like electromagnetic sensing, sensing what’s happening in the em spectrum.
We were using AI to fuse all of that information together and then provide iCare an integrated local operating picture is what we called it to the, the human commander to say, this is a bit unusual. You might wanna have a closer look at that. So it’s a way again, of helping using AI to help the. Do better at their role enabling that human outcome to be better than it might be otherwise.
I mean, what, what, yeah, that strikes me as
a, you, a pattern of life study that a soldier would do from a, from a Sanger or from a century position.
So that, yeah. Yeah. That’s yeah. Okay. Delete
your port cabin and all the screens we’d have hundreds of bases over, you know, the conflicts we’ve been through a soldier, stands on a century position and looks out for people, but he also looks out or she looks out for the pattern of life.
Particularly in a counterinsurgency cast stand, is this normal mm-hmm and this sentient, you said
a sapien SAP SAP.
That’s right. Sapiens. Yeah. Can as can do that for you. Yeah. Or can, can give you a bit of assurance
on top of that. And what, what I love about sapien so much is, is what’s called an open architecture.
It sounded really boring but it’s actually really important. And why it’s so important is that it defines all of. Standards, all the interfaces that allow us to plug together lots of different industry providers solutions. So, you know, we could have a, a really world leading sensor from, you know, say kinetic or a company like that.
And then we could make sure it can talk to another world leading sensor from Don, no north of Grumman or, or, or, or Lockheed Martin or and. For me, that’s where it gets so powerful. You’re not just using AI in one little narrow sort of application and one little sensor you’re using AI to make all of these things talk together.
And that I think is where we get some massive competitive advantage for our people. Cause it can help us get after things like pattern of life. It can help us. Take the absolute best technology from whichever company it comes from and make it all work together. That’s really hard, but what an important problem,
but there’s also something else on this.
Isn’t there that, that I’m, I’m guessing knowing DSTL for years, that, that you’ll also be looking at, which is lots of these. Techniques processes that AI uses could be potentially D they could run in the way of the way that we want wars to be fought in the future. Right. They, they run against our morals and our ethics and all of that.
So, so I’m imagining that part of DSTL has this program that says, you know, we are going to be governed by these following standards, right. These ethics and that works alongside. So what, what I’ve seen a lot in academia is that the, the intellectual discussion about how you’re gonna use. AI systems is somewhere behind the technology and, and it, and it lags.
So you’ve got these great leaps in AI and who can read data and you know, what the database could be used for, or just database is being released without that argument of, should it be released? Should it not be released how it’s gonna be used. Now I’m guessing here that there is a sort of, I don’t know, it’s part of your role looking at the, you know, The, the compliance to a bunch of, you know, codes, morals, ethics, behaviors that we see, this is how we want AI to develop, right?
So I think this is absolutely critically important. If you say there were AI to, you know, the average person on the street, they start worrying about their job. If you say AI in defense, they start worrying about the Terminator and, and, and killer robots. And for me, understanding how we can responsibly and ethically harness.
AI for the war fighter in a way that makes the world a better place and a safer place really is like a critical challenge for our generations. So I feel really motivated as a scientist, working at the STO to try and make sure that we are. Making good choices about how we apply AI to what we do.
So we think really hard about, you know, the misuse, what some of the downsides might be. You might have seen that in June the mod published a document called ambitious, safe and responsible, which described the five ethical principles. O D well, I didn’t
read it make, so you’re gonna have to run me through what
Can you remember that? The, oh, you’re gotta put me on the spot now, but it’s all about making sure that we don’t build AI systems that are biased. Making sure that we reduce the overall level of harm and use AI as a, as a, as a, as a force for good. It’s making sure that AI systems can still be account.
A human commander still ultimately has to be accountable for the, for the, for the, for the military effects that they are that they are applying. There’s a big focus on trustworthiness. So making sure that AI systems can be trusted by the commander, but also trusted by the public that ultimately we, we, we, we all serve.
So those ethical principles really. Run through all of our work here at D SDL. What we are increasingly doing is all of the scientists here are working in multidisciplinary teams. So I’ve got teams of. Software developers. I’ve got teams of machine learning, engineers and data scientists. But then what we’re doing is, is budding them up with like AI ethicists who can be working with them right from the outset, not like coming in as a sticking plaster at the end.
But really like working with them in partnership. We also work with like user experience designers and human factors, experts to make sure that we. Really put the human at the center of all the systems we build. We also work really closely with the military domain experts. They’re really, really important cuz they help the scientist engineers understand how, how this can help.
So yeah, an answer to your question ethics and responsible AI, it’s absolutely a heart of our approach. And then we’re trying to use this kind of multidisciplinary way of working to really make sure that we design systems that. And that, that, that really make the world a better place. It’s interesting.
At the start of that, you talked about bias though, cuz cuz I, you know, having read a bit about AI, that there is a, there is a increasing worry from some academic circles that, you know, bias in AI, even from right then, you know, the database that you collect at the start will give you a different answer.
So if you go back to cats, mm-hmm , you know, if, if it was just. You know whiskers and nose and ears, then, you know, 50% of where you gotta be dogs. And if you put that into a C2 system or an ISR system, you could come up with the wrong answer. So, so what are the big risks in AI? If, if we, you know, if we’ve, if, if we are really thinking about bias and our level of trust and accountability, what are the, what are the real risks that we’re left with with AI that, that keep you up at night?
Yeah, so I think bias is a real challenge and it’s almost inescapable in. No matter how hard we try bias is always baked into our data sets. And if the way the machines learn is through some kind of data, which is inevitable. You know, it’s almost impossible to completely remove that bias from the system.
But the thing that gives me hope is that we’re not, we, we shouldn’t be holding our AI systems to an unachievable standard. We are all biased. Every human. Has his or her own biases and that, you know, that’s dependent on, you know, how we’ve grown up, what experiences we, we, we we’ve had in our lives.
Well, I
think it goes back to what we talked about earlier. You’re trying to teach common sense and that is part of what bias does, isn’t it? Yeah. The heuristics we talked about allows the human to quickly identify a cat because a biases teach it quickly. That’s probably a cat in me. It’s got four legs, a 10, one point a ears.
Yep. But actually it might not be, it might be something slightly different. Yeah. So, but there’s a real tension there then isn’t
it obviously. Useful. What, what, what I think helps though, is that I can measure bias in an AI system. If you had to put a percentage on me of how biased I am a different thing, how on earth would you do that?
But I can actually write down a percentage of how biased a a machine learning system is. So a very well known and really unfortunate example is the fact that facial recognition doesn’t work as well on people of different. Origins, because a lot of the faces in the training set have been white faces.
You know, that is an unacceptable outcome, but the good thing is I can sit down and I can work out repeatedly. How many, how many times the AI system gets it wrong? If I can measure it, I can fix it. So for me, when people talk about bias in AI systems, it’s a really, I. Problem. But I worry that people sometimes attach a sort of a, an unattainable gold standard of this AI system can have no bias in it whatsoever, but not recognizing that actually we are biased as humans.
And, and for me, a lot of my time is spent trying to kind of manage the hype if you see what I mean.
So the analogy that I like to draw is relating to kind of autonomous cars and how. Actually, I think what we’re going to see is the gradual introduction of more and more AI, more and more autonomy into the still human led process of driving a car. And you know, it might be helping you stay within your lane.
It might be Free priming your brakes, or even automatically activating your brakes if you’re about to get into, into a crash. And I think what we’re going to see in the automotive sector is the kind of the gradual kind of introduction of more and more ironed autonomy into the car driving process.
And it might be in, you know, I don’t know how many years, but at some point, you know, we’ll go to fully self-driving cars, but I think actually it’s happening a lot slower than people expected because of those trust issues. And. Some of the fatalities we see, I think we’re gonna see exactly the same thing in the military domain.
And a bit like we were talking about in a military headquarters, how, how can we begin to just improve the quality of life for offices in a, in a military headquarters initially and some fairly small focused tasks and then incrementally getting more and more AI into the system till, you know, maybe in.
10 or 15 years, some of those things you mentioned might be possible, but what I don’t want to do is to overinflate expectations, get people thinking that AI’s some kind of silver bullet or kind of magic that can, that can solve all of their problems. It’s a really powerful technology, but we need to, we need to take baby steps to get to the big changes.
It
it’s at the start of a military career, right. It took a long time for the military to trust me to do anything. So I imagine general . I mean, you’re absolutely right.
And I think about this a bit like you know, how we’ve introduced flight into military operations, how we’ve introduced precision guarded weapons, how we’ve introduced kind of the maneuver risk type approach.
It will take time to figure out how we can really incorporate AI into our ways of working and our kind of military trade craft. Is not gonna be something that’s gonna happen, you know, overnight in a year or two, we can, we can definitely get some quick wins, but this is a, a marathon, not a sprint. This is something that is incredibly profound in terms of its potential impact on, on, on, on, on the military domain.
And we need to be in this for the long haul and it’s so, I mean, I, I, I just think I, I feel so admiring of all of the experimentation and all of the user. Stuff. There are people all over the mod who are just rolling their sleeves up and seeing what they can do in this space. And it’s that kind of spirit of kind of experimentation and trying and learning and failing and, and, and things.
That’s going to get us the outcomes that we want. And. You may have also seen that what we are now doing as part of the defense AI strategy is establishing a defense AI center. And that’s really trying to support all of those different local innovators around defense and make it easier for them to make changes in their area.
But that that’s the
essential bit, right? Cause you’re talking about, you know, already we have all these sort of AI ML systems that are already using maybe the same databases in a lot of circumstances. They’re all putting it together. Maybe coming out with different answers, everyone’s developing.
Sometimes in stove pipes, someone’s gotta be able to draw them together so they can exchange so they can be used to validate and verify so they can share knowledge and understanding. I mean, that that’s gotta be the, the,
the Keystone, right. And that is exactly what the defense AI center or the date as we call it has been created to do so.
The date is a partnership between UK strategic command and defense equipment. And. DSTL and then working with all the different frontline commands and, and, and, and top level budgets across defense. And exactly what you just said is what the deck is trying to do. It’s not about trying to do all the defense’s AI work in one place or, or, or one, one one building it’s about trying to build a community across, across fence.
It’s about trying to share best practice between different areas. It’s about trying. Come up with common solutions that everybody’s banging their head against and figure out how we can solve it once for everybody. And I think perhaps most importantly, it’s about being the, kind of the visionary champion for AI within defense, really helping defense understand what AI could could do for it.
So as well as my work here at the SDL, I’ve got, I’m leading the SDLs contribution into the, into the. And so it’s a really exciting time to be working in AI, in defense. It gets back to that,
that, that, just that talk about the, you know, the, the central pillar for putting all this together, but it gets back to one of your points about trustworthy.
Because, you know, if you’ve developed a system with AI, right? The looks at databases, you know, you understand you you’ll trust it, right. Same way. Rusty would trust, you know, some of his, his section tune a company, you know, you would trust those people, you know, which are the good, which are the bad you wish to take with the pinch of salt.
I would trust, you know, different missile systems or radars or sales. You know, we have a different relationship with trust, but then to suddenly give. Frosty a completely new AI system going, this is gonna give you, you know, your answers to your ISR. There’s, there’s a, there’s gotta be a point in there that that trust becomes a really big part that we’ve gotta overcome rather than just, you know, I see a real radar return.
That’s where it is. You get to different. I, I
couldn’t agree more. And for me, it’s about this kind of incremental process that I was that I was describing earlier. So for example, We do an awful lot of experimentation work in the S STL and working with our partners in, in, in frontline commands. And to make that work we use need to use what’s called agile techniques.
You might have heard of DevOps or dev sec ops. The new sort of buzzword is ML ops machine learning operations. But for me, what’s so important about that is you need to. The people developing the systems, the scientists and the engineers that can build these systems. You need to get them alongside people like frosty and kind of really understanding what are the problems that you are encountering.
What are the things that would make you trust this system? What are the things that would, that would, that would, that would be a problem for you? How can we make this work for you? How can we do that kind of support? And that, that lops approach is become the kind of the industry norm in the civil.
It’s how banks, it’s, how insurance companies, you know, that’s how everybody delivers AI into operational use. And so what we’re trying to do with the defense AI center is take some of that industry best practice and get it working for us within defense. So, you know, I know you know, war fighters are, you know, they’ve got a huge task on their hands, but if there are, you know, a little bit of time that we can, they can spare to work with the scientists and engineers to help.
Understand how the systems that we are building can be made to work for them, where we can get our hands on real data that is like gold dust. Those are the things that are gonna help us build those, those trustworthy systems. And what we’re really trying to do is, is harness the best and the brightest mines that we can get anywhere in the UK and get them working on our, on our problems.
So in DSD, we’re working with some really like world leading. AI industry companies. We’ve got links that we’ve got to some of the best universities in the world. We wanna get all of that brain power solving your problems. So that, I think that kind of agile approach working really closely between the scientists of the end users.
That is the way that we’ll get to that kind of trustworthy state that you mentioned
interesting, that, that you focused there on the UK. And, and you know, when you look at the UK’s budget government spending on. Big right. But, but it’s tiny compared to the us and even India, Israel has been, has been working with ML systems for the IDF, the Israeli defense force for a, a long time.
Right. Operationalizing it very, very, you know, frontline focus, maybe even without some of the more. Without some of the ethical and moral restrictions that, that you might be, you know, that we might have to work with here. Right. And that’s, and that’s fine. So how much of this stuff do you do that is just UK and how much is done in corporation with others?
How are you able to pull in like the big lessons from India or Thailand or Australia or wherever? Is there a sort of five I’s NATO working groups that you relate this to? Or is it all very stove? Pipe, cuz this stuff really is just, it’s still behind the curtain, right?
So adopting AI is absolutely a team sport.
And if we think that we’ve got all the answers here in, in mod or, or of the SDL, we’re kidding ourselves. And I spend a huge amount of my time building relationships, doing technical exchanges with. For companies with international partners. So we obviously do a huge amount of work with the, with the us and we have joint teams that are developing solutions.
Also with the or the tri later MOU that’s been established recently, there is an AI working group under that initiative. We have a, a five eyes experimentation thing. So that contested urban environment experiment, I told you about that was a a big five eyes experiment. I think we had over 30 or 40 systems that came from all of the different international partners.
And then finally, we’re I think trying to expand our approach out to some of the international partners that maybe we don’t traditionally work with quite so much kind of beyond the, kind of the NATO nations. For example, I’m part of something called the AI partnership for defense, which is a 16 nation initiative that involves the, like to sort Singapore, Japan, South Korea Israel, part of that actually, as well as some of the more traditional kind of NATO partners that you expect to see.
So it is massively a team sport. The other thing I just quickly wanna say on that is that we are. Pleased with how engaged some of the big technology companies are being with defense. They want to use the benefit of all of their experience to help us get the right outcomes for AI within defense.
So as well as all the normal kind of defense primes that we’re working with, we’re also talking to the big tech companies and trying to draw upon their huge resources to help us get after this problem for the. I
got, I got two final questions. First, Steve one is one is what keeps you up at night, you know, in, in AI with DST, what keeps you up at night?
And you sit there going, I mean, is it, is it the commute to work? Is it budget? Is it, how far are you allowed to run, you know, your lunch? So what is it that, that keeps you up at night? What worries you most about, about this?
Maybe a couple of different answers to that question. So the, the first answer is maybe that.
Big generational challenge. I could imagine some futures where AI is a, is a wonderful force for good. That really reduces the overall level of harm in warfare. That saves lives that helps our commanders make better quicker decisions. That makes the business of war fighting, you know you know, less, less, less, less, less You know, bad for, for, for, for, for, for the world.
But I could also imagine some, some horrendous scenarios, particularly, for example, like information warfare and like misinformation flying around. So for me, I feel really responsible as a scientist and engineer, trying to make sure that we take the right steps, get us towards that kind of outcome.
What we could see is a kind of a race to the bottom. Countries kind of, you know, sort of taking more and more steps that really push us down a, a path. So I, I worry about that. That feels to me like a, a, a, a really big challenge. The second thing I, I worry about is what we call the AI paradox. So it’s quite easy to do, or it’s not easy, but, but it’s easier to do an experiment in a lab and shape doesn’t AI system look great, but then there’s a big difference between that fantastic experiment in a.
Rolling out an operational capability to tens of thousands of military users. So how can we get more AI into the hands of war fighters in a way that they can trust it and, and, and roll on it? So that’s the second thing I worry about. And then the third thing, and the final thing is about best and the brightest brains.
AI is one of the most sought after skills, anywhere in the world. At the moment. We need to make sure that we can attract best and the brightest people to come and work on some of these really important problems. If we’re gonna solve some of these things, we need the smartest, the most committed, the most dedicated people who can come and work with us.
So. For me, making sure that we’ve got access to the best talent that we can. And DSTs got a fantastic role. And we, you know, we’re working really hard to bring all those bright minds together, but the more bright minds we can get solving our problems to give operational advantage to end users the better.
So, yeah, those are, and I
guess that’s that, that links in ply to my final question to you, which is, listen, you, you know, a lot about AI, you’re in a, you’re in an industry, you know, an area of industry that. Seriously in demand globally. You could walk out of here into, you know, something with rockstar wages, which is not something that government pays civil servants and, you know, that’s the fact of life right.
Industry would, would desperately love to have get their hands on you and, and pay you well. And you take your family on, you know, weekend breaks to the say shows wherever it would be. I mean, you would have like a really good, like what keeps you at DST? What keeps you working for the government? What is, what is that thing that.
Yeah, I’m staying here. I’m sorry, darling. We’re going on a leaky 10 in Cornel again. but what is it that, that keeps you here? So
I love what I do and for me I, what matters to me is using my expertise to make a difference, to save lives, to really you know, make sure that that, that our war fighters have got the best port they can possibly get, you know, Ask our armed forces to put their lives on the line, put themselves in harm’s way to keep us all safe.
If as a scientist, I can do something that can, that can help them. I feel like that is my moral responsibility to do that. And, and, and frankly I get paid enough to have a, a good quality of life. I’m not getting rock. Wages, I couldn’t afford a, maybe a, a leaky buttons thing rather than a leaky tent.
But for me, what’s much more important is feeling like I’ve got a purpose in, in, in what I do feeling like I’m able to really be working at the bleeding edge of, of, of, of, of science and engineering. So for me, I’d just get so motivated. I’ve worked in university labs in the past and. The STL. I get the chance to see my science being put into action in the real world.
And that is priceless. y a lot, particularly about bias and trust.