The transformative capacity of artificial intelligence (AI) for defence and security is incontrovertible if it can be exploited. The 2023 Command Paper highlighted that AI is, or will become, a strategic enabler for the UK MOD.
One of the more demonstrable impacts AI will have on Defence will be to speed up the observe-orientate decide act (OODA) loop to enable faster decision-making. Automating tasks will increase human capacity by allowing insightful access to greater quantities of information, increasing operational tempo. But AI is not just an enabler. It will become a domain in its own right and a contested one at that. As AI becomes more ubiquitous, so will the capabilities that are intended to trick and manipulate it. The desired effect is to break the trust that a human will have in AI, removing the advantage that battlefield AI provides.
This article will outline the threats to AI on the battlefield and discuss the principles that are essential to building AI systems that can be trusted by commanders.
Exploiting AI in Defence
Before any AI can be fielded, it needs to be developed and deployed, which, in turn, requires Defence to be ‘AI ready’. Establishing trust in a fielded AI system starts with organisational approaches to AI that seek to maximise the probability of deployment. This includes effective upskilling personnel and AI-optimised architecture (i.e., compute where it needs to be, efficient bandwidth, etc.). It also requires adapting risk management approaches and a pivot towards the more Agile approaches that support continuous iteration and continuous deployment (for reasons that will become clear).
The UK AI sector was worth £15.9Bn per annum and in 2022 the global corporate investment in AI exceeded £90Bn. Yet, despite this investment, globally, a staggering 85% of AI models falter upon deployment. In comparison, the MOD has set aside £6.6Bn over 5 years for research into artificial intelligence, AI-enabled autonomous systems, cyber, space and directed energy weapons. If we conservatively estimate that the MOD spends £250M on AI-related technologies, then Defence cannot afford to only have 15% (£32.5M) of AI projects be successful.
Any badly performing or unsafe AI, anywhere in the organisation, could be considered a bad investment, and should be considered an operational risk. Adopting innovation principles and evolving procurement approaches can de-risk some projects, but more can be done to establish trust during the early stages. For example, technical benchmarking of AI performance during procurement would be a more comprehensive approach to ensuring that AI technology is suitable for a requirement.
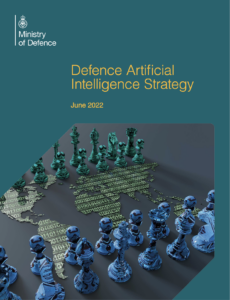
Integrating AI into the operational landscape is where, arguably, Defence has even less margin for error than industry. It is here that the safety, ethical, and legal considerations surrounding AI will cause a significant tug-of-war between adoption (to realise the benefits) and caution (to manage the risks).
To realise the benefits of AI, those wishing to adopt need to ‘win’ the debate by showing the benefits, but risk still needs to be understood and managed. Evidence of performance can inform the risk-balance judgement building confidence that a system will perform. This isn’t isolated to AI. Every piece of equipment is tested through its development and subject to evaluation. This process defines operating conditions, capacity, identifies vulnerabilities, and establishes limitations.
Consider the role of a remote vehicle equipped with multiple sensors for a find/fix/track mission. In an AI-enabled autonomous role, those sensors will need to detect objects of interest, cross-validate signals, and deliver an output back to a human operator. A human is detached from the information loop, and is alerted when the system detects something of note. In this mode, a single human can operate multiple such systems simultaneously.
Before this point, significant effort is needed to assure performance. The performance of each AI module needs to be determined with both expected and unexpected inputs, individually, and as an ensemble. The objects that they are designed to detect need to be trained – and retrained – into the system requiring labelled training data. As systems evolve then it needs to be updated and upgraded rapidly, possibly within days. When retraining is completed via reachback bandwidth needs to support vast quantities of data.
Tricking AI
It’s essential to remember that AI doesn’t exist in isolation. At its core, AI is driven by data and intricate models, which form a chain vulnerable to external influences. When trained, the models can be accessed, exploited, and contested; underscoring the imperative for security and a holistic understanding of AI’s role within the broader military ecosystem.
It is possible to systematically trick and deceive an AI system meaning we cannot simply see AI as an enabler. Rather, we must see it as a domain in itself, a domain that needs defending and dominating. Poisoning training data or perturbing the live input data can cause a model to fail; the potential impact on fielded AI systems should be clear. New camouflage techniques will evolve to render AI detectors ineffective. Large numbers of perturbations can ‘jam’ or overwhelm an AI system. Evolved techniques can force an AI system to misclassify, declassify, or falsely classify an object.
This technology is distinguished from traditional security challenges in that the weaknesses that are exploited are fundamental features of that system. These features originate from either model or data, or the training of the model with the data. Direct access to either isn’t a requirement. Pre-existing vulnerabilities can be ‘baked in’ during training; they remain because they are rarely systematically identified or mitigated during build. They cause unpredictable behaviour, strange predictions, and there are many ‘novel’ examples.
Recent experience
The USMC was involved in an exercise which tested the ability of a perimeter object detection system to identify incoming personnel. The value of such a system is to reduce the workforce burden of base security. AI was trained and then tested. It was then subjected to some novel counter-measures. Dressing up as trees ensured a group of soldiers were not detected. Hiding under a cardboard box and roly-polying to the target had the same effect. This example almost certainly involves a basic proof-of-concept system that is easily fooled, however, sophisticated systems can be subjected to sophisticated deception.
This concept – and threat – is uniquely relevant to the field of AI, and for militaries seeking to adopt AI. Its intended effect is to undermine the trust users place in intelligent systems and erode confidence in AI’s decision-making integrity. If an enemy can manipulate the AI system to produce inaccurate results without detection then our decision-making calculus is corrupted. This also creates a scenario where even if the AI system is functioning correctly, its outputs may be second-guessed or disregarded due to fears of manipulation.
This uncertainty can hamper decision-making and coordination and may even render the AI system useless if trust is eroded. If an AI model is not trusted to augment a human then that human-machine team is slower, less efficient, and less informed. While users might traditionally be wary of system breaches, adversarial AI poses the more profound risk of side-lining the adoption of AI and exploiting its broader benefits.
Reliable, robust, resilient
Trusting an AI model should hinge on establishing the reliability, robustness, and resilience of a system. Exploiting AI hinges on consistent and reliable performance, especially in unanticipated scenarios. Robust testing and validation under varied conditions is vital to ensure the system’s adaptability and reliability. Considering this as an afterthought of AI development reduces the chances of successfully deploying a system safely. We don’t build bridges without understanding the characteristics of the materials and how they fit together. Nor should we for AI.
Reliability, the consistency with which an AI system produces accurate and dependable outcomes, involves rigorous testing, continuous monitoring, and validation of models across diverse datasets and scenarios. An effective CI/CD pipeline can constantly update and retrain models based on the latest data or evolving operational context (for example a new or adapted piece of equipment), ensuring that the AI system remains dependable. Establishing reliability is crucial for gaining trust, as users can be confident that the system will perform consistently, irrespective of the variations in input or context.
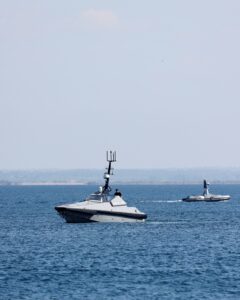
Robustness, the capacity of an AI system to perform effectively under varying conditions or when facing perturbed inputs, means prioritising the system’s ability to handle adversarial attacks, out-of-distribution inputs, and other challenging scenarios. Techniques like adversarial training, where the model is trained with intentionally perturbed inputs, can improve the AI’s resistance to malicious attacks. Moreover, stress testing a system to breaking point can determine failure points and establish operation envelopes. Trust in an AI system’s robustness means that stakeholders believe the system can handle unexpected or extreme conditions without catastrophic failures.
Resilience, the ability of an AI system to recover and adapt from unexpected disruptions or challenges to its functioning, focuses on the system’s ability to recover and adapt following disruption. Monitoring tools alert teams to system degradations or failures, triggering automatic recovery protocols, defensive techniques, or human intervention. By preparing for uncertainties and building self-healing capabilities, resilience ensures that AI systems can bounce back from issues, establishing trust in their long-term utility and stability.
When users observe an AI system consistently performing as expected, their trust in the system grows. This trust, in turn, boosts the AI’s performance, as users will confidently integrate the system into decision-making processes.
Maintaining trust
Establishing trust isn’t a one-time event, nor is it established by creating robust AI alone. Rather, it is a continuous journey demanding both systemic modifications and a shift in mindset. To trust AI requires that we habitualise our approach to its development ensuring that at every stage the system aligns with core principles.
Trust is enhanced by organisational approaches to concepts such as Transparency. The more a user understands how AI works, the more they are likely to trust it. Systems that function as “black boxes”, AI systems that obscure their internal reasoning, are harder to trust because their processes are obscured. Moreover, allowing human oversight, can bolster trust in the predictability of AI decision-making. When human users can anticipate and understand how AI will react, it engenders confidence in the system’s reliability.
Harnessing low-risk AI applications while individuals engage in upskilling, and as Defense adopts AI technologies, can contribute to the establishment of trust. Focusing on low-risk AI use cases, such as automating routine tasks, enables users to experience the value of systems firsthand, fostering a sense of familiarity and trust. This can serve as a gateway to higher risk use cases of AI including autonomous vehicles and intelligence where AI can have a substantial impact.
Conclusion
The harmony of humans and AI promises a Defence paradigm where efficiency meets reliability. But only if that relationship is built on trust and the AI is properly protected.
Even the most advanced AI system’s efficacy is contingent upon human trust. In high-stakes military operations, trusting in automation isn’t just about believing a system will work, but that it will work under pressure. As Defence pivots towards an AI-augmented future, fostering this trust becomes as critical as addressing the technical challenges themselves. If a decision-maker is uncomfortable with an AI system, they might choose to ignore or override its decisions.
An emphasis on crafting robust AI systems is paramount for fostering trust in artificial intelligence. The early stages of AI/ML development are pivotal. A proactive approach doesn’t just enhance functionality, it acts as a shield. By pinpointing and addressing AI’s potential failure avenues early, we not only minimise unintentional errors but also fortify the system against vulnerabilities that adversaries might target.
Maintaining trust is as important as generating it. Continuous performance assessment, underpinned by metric tracking, becomes essential to ascertain the model’s enduring competence. This relentless scrutiny, spanning the lifecycle of the AI system, is a bulwark against complacency. By vigilantly monitoring the system’s health, any signs of impending failures or aberrations can be detected and addressed promptly, thus ensuring that the AI remains a reliable ally, and the trust vested in it is continually reaffirmed.
Ben Fawcett
Ben Fawcett was a Royal Navy staff officer for nearly 14 years serving in a variety of intelligence, operations, and innovation roles. He now works at Advai, a start-up developing tools for AI robustness, and collaborates with Defence operators to understand how AI can be safely exploited on operations.